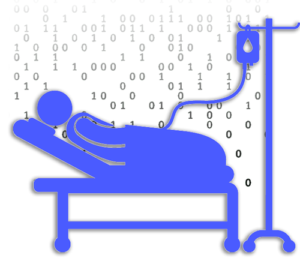
Recently, a group of former patients of the University of Chicago Medical Center (UCMC) had filed a class action lawsuit against the Medical Center, the University of Chicago and Google1. With new technology in privacy preserving computing now available this data exposure (and negative publicity) didn’t need to happen.
Without commenting on the merits of the case, the crux of the argument for the complaint is that Google violated the Health Insurance Portability and Accountability Act (HIPPA) patient security protections by receiving electronic health record data from the UCMC that could reasonably identify who the patient was, including date stamps for the patient’s admission and discharge, as well as free form text via doctor’s notes.
The hope is that Google, the undisputed experts in tailoring the digital consumer experience, would use the type of data obtained from UCMC in their models to help generate better predictive outcomes and thereby increase patient safety, improve care, reduce invasive surgery and decrease health costs.
It sounds like they did. In a paper published in early 2018, but not yet peer reviewed, Google claims they achieved ‘vast improvements’ over existing models and perhaps most importantly, were able to be more predictive of patient deaths a full 1 to 2 days in advance of current models. That is 1 to 2 more days where a doctor or team of specialists might be able to try new approaches in saving the patient’s life.
The complaint does get a few things right:
To effectively train machine learning models, researchers need access to a lot of data.
The concept of longitudinal data collection and research is nothing new. One of the most popular, the Framingham Heart Study that began in 1948 and is still ongoing, tracked over 5,000 patients and led to critical advances in our understanding of heart disease.
Google previously launched an initiative called Google Health in 2008 that allowed patients to voluntarily opt into sharing health care information about themselves like their existing conditions and medications. This would allow consumers, at no charge, to have a merged health record to alert them of possible health conditions or drug interactions.
As you might have already guessed, large droves of consumers willing to volunteer very sensitive personal healthcare information never materialized and Google Health was shuttered in 2011. This exercise did presumably give them a window into how fragmented the patient electronic health record system was. Health care provider networks have their own record formatting and vary as to which data points they are willing to collect. Doctor’s notes and prescriptions are still often handwritten. The sheer process of cleaning and normalizing the data from each network for predictive modeling likely took up the majority of the time they spent on this project. That’s likely why they wanted to work with just two hospitals on this project2.
The combined data sets produced over 46 billion data points from over 200,000 patients. In the world of machine learning, more (good) data beats better algorithms and a wider sample size will produce more reliable predictive models. Recent developments in deep learning and artificial neural networks have allowed these systems to handle this messy data and even detect incorrectly labeled data.
Your healthcare data is likely already being made available to a number of third parties.
Every day a great deal of data is generated derived from software and hardware and how you engage with it. You generate enormous amounts of data and might not know it; from the apps you use to how far you read into a news article to what you search for. This in turn allows companies like Google to optimize your user experience and create advertising products.
While wearable consumer apps that track, for example, the amount of miles you have walked over the course of the day are not covered by federally regulated privacy protection, patient data in the form of electronic health records is.
The HIPPA Privacy Rule refers to this sensitive information as Protected Health Information (PHI). Basic identifying information about someone, like their name, age and social security number in conjunction with their medical history or their ability to pay for medical services would be considered PHI. A report showing what the average age of patients was over a time series, though, or the number of male versus female patients would not be considered PHI.
PHI data in an anonymized format is a multi-billion dollar industry. Records from pharmacies, medical practices, insurers, and government entities send some portion of their records electronically to data brokers, like Optum and Truven Health Analytics, who then generate insights from the data.
So the odds are, your data is in some way being sent to one of these data brokers and it doesn’t require your permission. Even the Centers for Medicare and Medicaid Services, a federal government agency, publishes anonymized claims data.
Deidentifying patient records or anonymizing them is not a good enough solution.
It has been proven that deidentified data points on anything from credit card transactions to healthcare records can be reidentified, often quickly, by trained data scientists with access to additional data points. A study conducted in 2000, for example, found that 87 percent of the U.S. population can be identified using a combination of their gender, birthdate and zip code. Another study used anonymized Netflix data to reidentify the subscriber. Data becomes far more valuable when you have the additional context that deanonymization provides.
Healthcare is a trillion dollar annual industry and it makes sense that new competitors would want to more aggressively enter that market. What if I also told you that these companies could have received the exact same information value from those patient records but without ever having received or having been exposed to the underlying patient data in a way that would address all three of the above issues?
That’s where Inpher comes in. Using our XOR Service, healthcare analysts could have performed the exact same functions on the data while it never left the hospital. No data transfer, no exposure to the underlying data or personally identifiable information and no loss of precision in the calculation3, which is critical when you’re talking about human medical diagnosis.
Inpher uses secure multi-party computation to protect data while it is in use. This means that healthcare AI models can be trained in a scalable way while at the same time keeping the data secure.
It is critically important that the level of trust between patients and their doctors, nurses and labs be maintained and their records kept confidential. Anything that compromises this trust will result in patients being less willing to provide helpful context on their medical issues, which will ultimately hurt the data driven advances that are being generated through the development and adoption of machine learning.
At Inpher we are making it possible to enable privacy protection on your health records at the same time as helping companies generate better, possibly life saving insights from that data. If you want to collaborate with us or have ideas on additional applications in healthcare, email us at [email protected]
Sources:
1 Matt Dinerstein, individually and on behalf of all others similarly situated v. Google, LLC, The University of Chicago Medical Center, and The University of Chicago (Defendants).
2 University of California San Francisco Medical Center (patient records from 2012-2016).
3 Currently up to six decimal precision.